Best Student Paper Award at AISTATS 2021
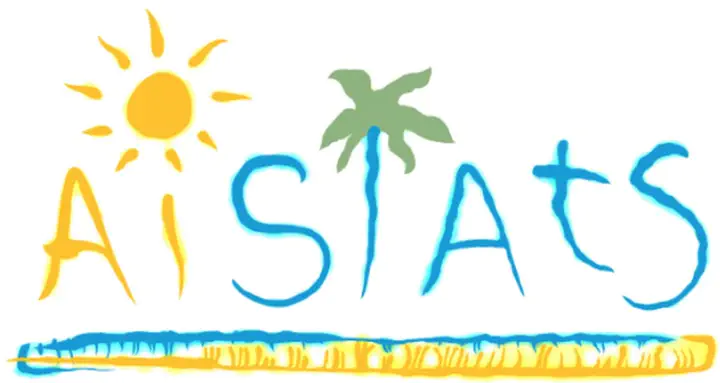
Congratulations to Viacheslav, Iskander, Alexander, Peter Mostowsky, and Nicolas for the Best Student Paper Award at AISTATS 2021.
- Viacheslav Borovitskiy, Iskander Azangulov, Alexander Terenin, Peter Mostowsky, Marc P. Deisenroth, Nicolas Durrande
Matern Gaussian Processes on Graphs
International Conference on Artificial Intelligence and Statistics, 2021
Gaussian processes are a model class for learning unknown functions from data. They are particularly of interest in statistical decision-making systems, due to their ability to quantify and propagate uncertainty. In this work, we study analogs of the popular Matérn class, where the domain of the Gaussian process is a weighted undirected graph. These domains appear in many interesting application areas, such as traffic modeling or protein folding.
Graph Matérn Gaussian processes can be trained using stochastic variational inference via inducing points, which enables their use in mini-batch and non-conjugate settings, and allows them to be trained using modern toolkits based on automatic differentiation. The graph Laplacian can be eigenfactorized, which enables one to define graph Fourier features, which accelerate computation and can enable graph Matérn Gaussian processes to be approximated even in cases where the graph is too large to fit in memory. The prior variance of the graph Matérn kernel can be chosen so that it reflects the structure of the graph.
You can read more about graph Matérn Gaussian processes in the corresponding blog post.