Abstract
We introduce a scalable approach to Gaussian process inference that combines spatio-temporal filtering with natural gradient variational inference, resulting in a non-conjugate GP method for multivariate data that scales linearly with respect to time. Through a natural gradient approach, we derive a sparse approximation that constructs a state-space model over a reduced set of spatial inducing points and shows that for separable Markov kernels the full and sparse cases exactly recover the standard variational GP. This leads to an efficient and accurate method for large spatio-temporal problems that we demonstrate on multiple real-world examples.Bio
Ollie Hamelijnck is a PhD student at the Alan Turing Institute and the University of Warwick, supervised by Theo Damoulas. Before this he was a research assistant on the London air quality project at Turing. His research interests lie in spatio-temporal Gaussian process and scalable inference methods.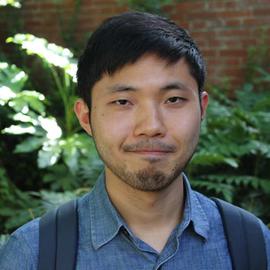
Senior Research Fellow (11/2020 - 07/2023)