Abstract
Gaussian Process (GP) models are a class of flexible non-parametric models that have rich representational power. By using a Gaussian process with additive structure, complex responses can be modelled whilst retaining interpretability. Previous work showed that additive Gaussian process models require high-dimensional interaction terms. We propose the orthogonal additive kernel (OAK), which imposes an orthogonality constraint on the additive functions, enabling an identifiable, low-dimensional representation of the functional relationship. We connect the OAK kernel to functional ANOVA decomposition, and show improved convergence rates for sparse computation methods. With only a small number of additive low-dimensional terms, we demonstrate the OAK model achieves similar or better predictive performance compared to black-box models, while retaining interpretability.Bio
Xiaoyu Lu is a machine learning scientist from Amazon, where she has been working on explainable models, attribution and graphical causal models. Prior to joining Amazon, Xiaoyu has completed her PhD in Statistics from University of Oxford, supervised by Prof. Yee Whye Teh. Her PhD research area include Gaussian Processes, VAE, Bayesian optimization, as well as MCMC methods.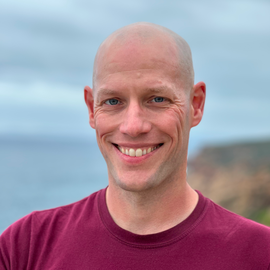
Google DeepMind Chair of Machine Learning and Artificial Intelligence