Abstract
Effective communication is an important skill for enabling information exchange and cooperation in multi-agent settings, in which AI agents coexist in shared environments with other agents (artificial or human). Indeed, emergent communication is now a vibrant field of research, with common settings involving discrete cheap-talk channels. One limitation of this setting however is that it does not allow for the emergent protocols to generalize beyond the training partners. Furthermore, the typical problem setting of discrete cheap-talk channels may be less appropriate for embodied agents that communicate implicitly through action. This talk presents research that investigates methods for enabling AI agents to learn general communication skills through interaction with other artificial agents. In particular, the talk will focus on my Postdoctoral work in cooperative Multi-Agent Reinforcement Learning, investigating emergent communication protocols, inspired by communication in more realistic settings. We present a novel problem setting and a general approach that allows for zero-shot communication (ZSC), i.e., emergence of communication protocols that can generalize to independently trained agents. We also explore and analyze specific difficulties associated with finding globally optimal ZSC protocols, as complexity of the communication task increases or the modality for communication changes (e.g. from symbolic communication to implicit communication through physical movement, by an embodied artificial agent). Overall, this work opens up exciting avenues for learning general communication protocols in more complex domains.Bio
Kalesha Bullard is a Research Scientist at DeepMind on the Game Theory and Multi-Agent team. Prior to joining DeepMind, she completed a Postdoctoral Fellowship at Facebook AI Research. Kalesha’s research is generally in the space of multi-agent artificial intelligence. It focuses on developing principled methods for interactive and reinforcement learning for artificial agents in cooperative multi-agent settings. Over the course of her career, Kalesha’s work has enabled learning in shared environments with both human partners (PhD) and other AI agents (Postdoc). Kalesha received her PhD in Computer Science from Georgia Institute of Technology in 2019; her doctoral research was in interactive robot learning and focused on active learning from human teachers. Beyond research, Kalesha has participated in a number of service roles throughout her research career. Most recently, she served as the Program Chair for 2021 NeurIPS Workshop on Cooperative AI. Among other roles, she has also served as an organizing committee member for the 2020 NeurIPS Workshop on Zero-Shot Emergent Communication, a Program Committee member for the 2020 NeurIPS Cooperative AI Workshop, and an Area Chair for the 2019 NeurIPS Women in Machine Learning Workshop. In 2020, Kalesha was selected as one of the annually awarded Electrical Engineering and Computer Science (EECS) Rising Stars, hosted that year by UC Berkeley.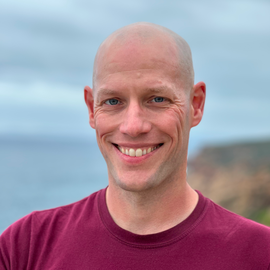
Google DeepMind Chair of Machine Learning and Artificial Intelligence